Do you research machine learning in Earth science contexts? Are you planning on attending the American Geophysical Union (AGU) fall meeting? Don’t miss your chance to submit an abstract to the Leveraging Unsupervised Learning Techniques in Earth Science Observations session.
Don’t Miss Your Chance to Submit
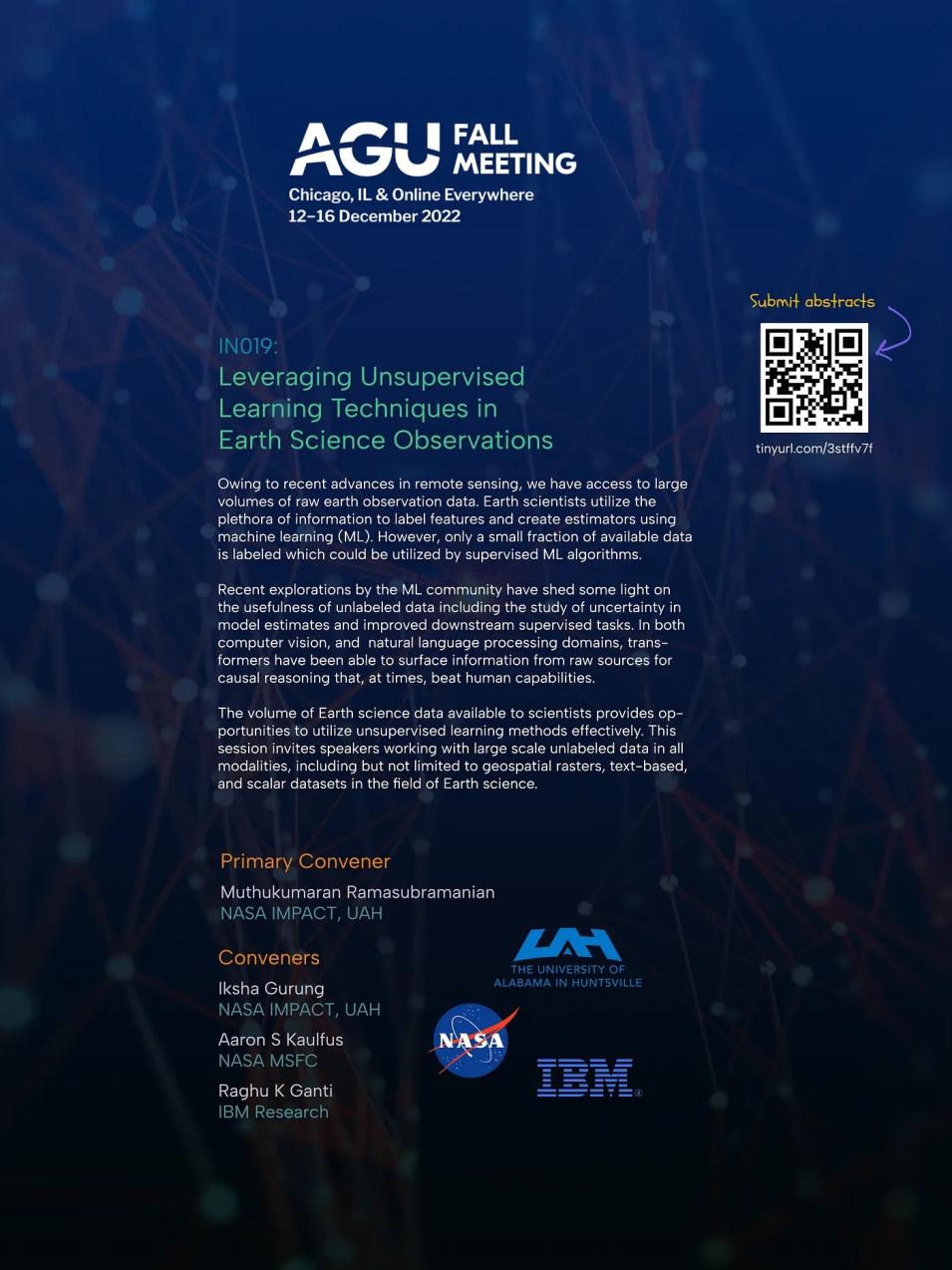
IN019 — Leveraging Unsupervised Learning Techniques in Earth Science Observations
Owing to recent advances in remote sensing, we have access to large volumes of raw earth observation data. Earth scientists utilize the plethora of information to label features and create estimators using machine learning (ML). However, only a small fraction of available data is labeled which could be utilized by supervised ML algorithms.
Recent explorations by the ML community have shed some light on the usefulness of unlabeled data including the study of uncertainty in model estimates and improved downstream supervised tasks. In both computer vision, and natural language processing domains, transformers have been able to surface information from raw sources for causal reasoning that, at times, beat human capabilities.
The volume of Earth science data available to scientists provides opportunities to utilize unsupervised learning methods effectively. This session invites speakers working with large scale unlabeled data in all modalities, including but not limited to geospatial rasters, text-based, and scalar datasets in the field of Earth science.
Learn more about or submit an abstract to session IN019.